Cross-Silo Federated Learning in Enterprise Networks with Cooperative and Competing Actors
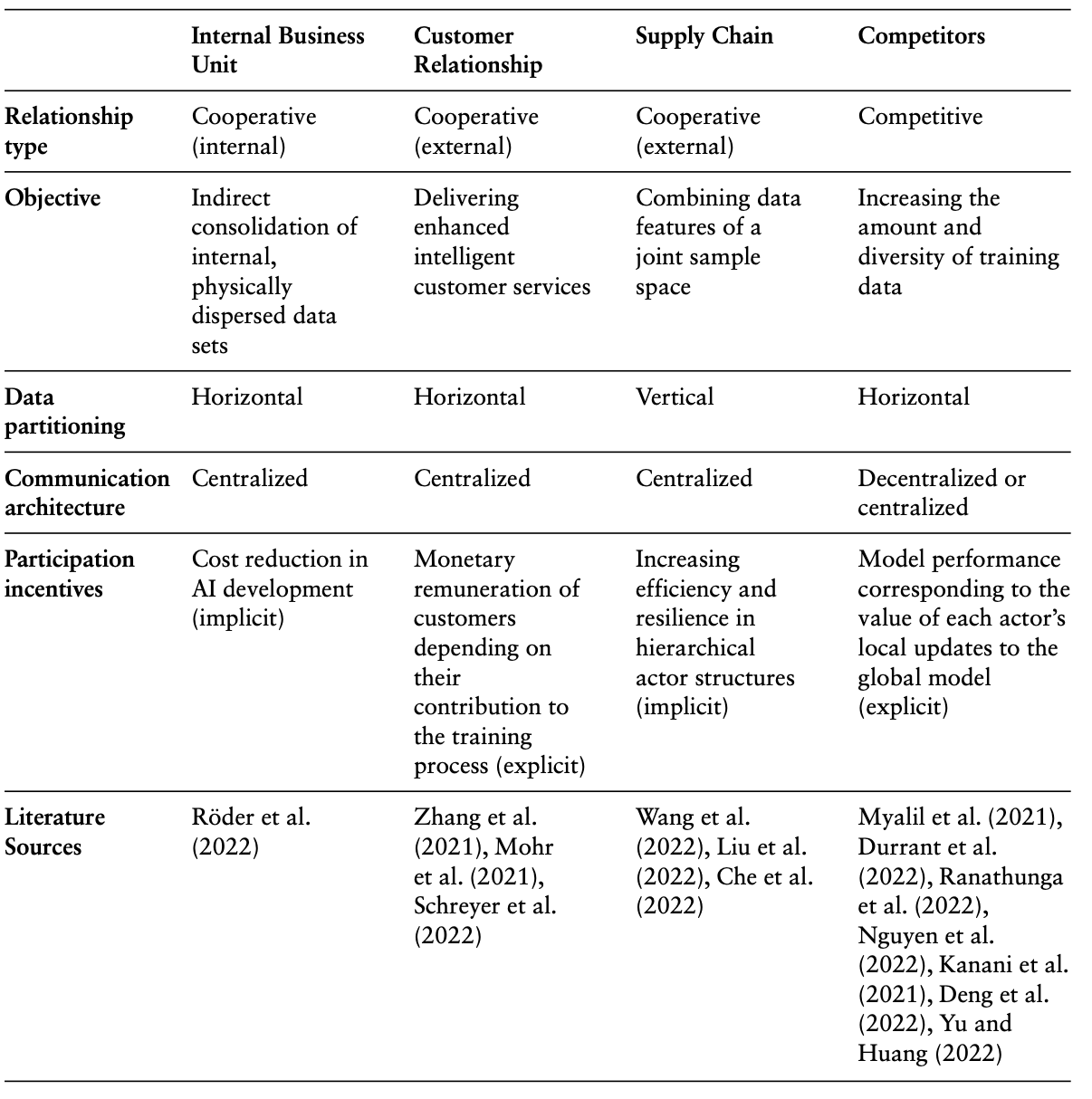
The performance and generalizability of AI-based enterprise applications depends on the quantity, quality, and diversity of training data. However, data usually exists in the form of data silos at individual sites. With cross-silo federated learning knowledge extracted from data silos that are distributed across multiple enterprises can be combined to improve the predictive performance of AI models without sharing and centralizing potentially sensitive raw data. The decentralized learning approach thus offers new privacy-preserving opportunities for cross-company collaboration, knowledge management, and the development of intelligent applications and services in federated enterprise networks. Since federated learning enables collaboration between both cooperating and competing companies, a literature review of application-based papers analyzes the differences in the design and strategic management of federated enterprise networks as a function of the actors‘ relationships.
Publication
Müller K., Bodendorf F. (2023). Cross-silo federated learning in enterprise networks with cooperative and competing actors. In: Christine Leitner, Jens Neuhüttler, Clara Bassano and Debra Satterfield (eds) The Human Side of Service Engineering. AHFE (2023) International Conference. AHFE Open Access, vol. 108. https://openaccess.cms-conferences.org/publications/book/978-1-958651-84-1/article/978-1-958651-84-1_25